Recently, the QRCA invited me to partake in a panel discussion centered on a burgeoning issue that plagues our industry: the rise of deceitful participants and false responses in online market research. This thought-provoking conversation has inspired me to offer additional perspectives on how technology and market researchers can work together to combat the issue at hand.
This unfortunate trend is heavily plaguing quantitative research projects given the ease with which AI-powered bot farms can flood available surveys. Though not as rampant in online qualitative research, we must be mindful that bad actors can be motivated by the larger incentives typically provided by qual studies.
As Chief Product Officer (CPO) at Recollective, I’m committed to combatting online qualitative research fraud as I believe it has a corrosive impact on our industry. Researchers can and should take proactive steps to ensure authentic responses underpin their insights and technology providers should offer tools proficient in preventing or identifying any unscrupulous behaviour.
My aim here is to suggest some effective solutions that can reduce the potential risks of fraudulent participant responses in your online qualitative study. Additionally, I want to draw attention to current and upcoming Recollective features designed to bolster the integrity of your research data.
How Fraudulent Participants Impact the Recruiting Process in Online Research
There have always been concerns about respondents' authenticity, especially when recruiting participants online or for online research. This is something that has existed even before the advent of artificial intelligence and I’m sure many researchers have seen this firsthand.
Laura Pulito, our VP of Research Services at Recollective, once encountered a participant who had entirely falsified her identity. Despite having a strong profile and responses during the online phase of the project, it was revealed that the participant should not have even qualified for the study once the in-home interview was conducted.
Such elaborate online deception is now more preventable thanks to the use of video questions in the screening process. Video submissions better approximate an in-person assessment and one can use them to confirm details like someone’s identity or their city of residence. A creative use of video might include asking applicants to show off a product they should already own or asking car enthusiasts to show their vehicle registration.
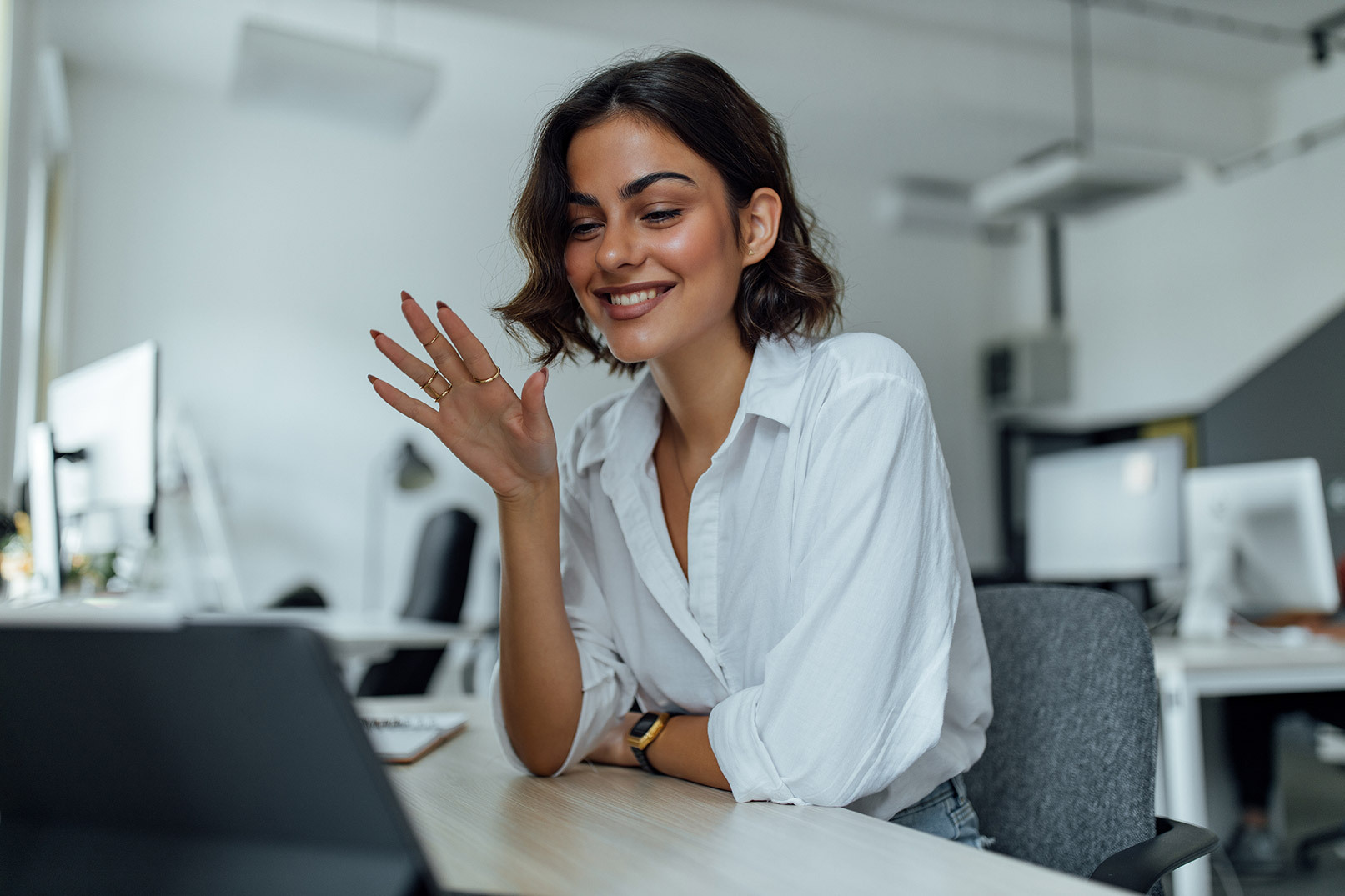
Having a more rigorous process at the start of a study will provide greater certainty while deterring less genuine applicants.
Once the study is underway, it may be beneficial for researchers to incorporate audio, video and photo-based questions. Audio recordings are simpler and offer more privacy than video, yet still capture the subtle details of spoken communication. These response types can help researchers evaluate if participants are genuine and engaged. It’s certainly more difficult to be oneself while reading a script. If there is sufficient time, carrying out live video interviews and group video meetings can be even more effective as they prevent participants from rehearsing their responses.
Ensuring Accuracy in Qualitative Research Due to AI-Generated Content
With tools like ChatGPT, participants can easily provide an AI-generated response as a replacement for their original thoughts. This risk is elevated in longer qualitative studies due to participant fatigue. Individuals may be motivated to find time-saving shortcuts while not actively planning to defraud the researchers. Regardless, quality suffers.
To combat participant fatigue and disincentivize the use of generative AI, I recommend building variety into your study design by leveraging Recollective’s many activity and task types. Enliven your studies by going beyond repetitive open-ended questions. Collect audio, webcam and screen recordings. Use image reviews, sort and rank tasks, fill-the-blanks and even grids to elicit commentary.
As responses arrive, ask detailed follow-up questions and encourage participants to connect their responses to personal experiences and previous contributions. Variety maximizes engagement while the structured response flow makes tools like ChatGPT less practical.
I also suggest leveraging a new feature Recollective offers to prevent participants from pasting external content into their responses. This can be enforced broadly for a study or enabled for select tasks.
The time of day and time spent on a response can also help identify suspicious behaviour. One should also be wary of photo submissions that appear to be professionally captured or AI-generated.
With regard to typed open-end responses, researchers should keep a watchful eye for common telltale signs of AI-generated content:
- Generic responses that lack emotion
- Absence of anecdotes and personal perspectives (e.g. minimal use of “I” or “we”)
- Uncommon word choices and over-hyphenation
- Excessive formatting like section headers, numbered lists, etc.
- Patterns in punctuation or sentence length
- Perfect grammar and spelling
- Americanized spelling in countries that use UK English
I recommend that researchers generate sample responses with ChatGPT to get familiar with its style of writing. Once you know what to watch for, most fake responses will jump off the page.
As the study progresses, I suggest viewing each participant’s profile page within the study given it displays all their contributions on a single page. Among other benefits, this view helps researchers spot inconsistencies.
If the unfortunate decision is taken to remove a participant from a study, Recollective allows all the contributions made by that individual to be immediately purged.

The Path Forward to Maintaining Research Integrity
Looking ahead, Recollective is considering the development of a number of AI and non-AI enhancements to strengthen our platform against fraudulent respondents:
- AI Detection: Although AI-powered content detectors can be defeated, they still provide a baseline of verification. In the future, we hope to highlight responses with a higher probability of being AI-generated.
- AI Generation: Administrators will be given the opportunity to generate AI responses while previewing their own tasks. This will save time while also demonstrating what type of responses AI will likely provide to their questions.
- Live Check-Ins: For live activities, we plan to enable a waiting room that permits hosts to conduct identity and tech checks before the meeting actually begins.
- Response Timing: We plan to enhance how asynchronous task responses are timed so we can proactively highlight outliers, such as those rushing through an activity.
- Session Analysis: Detailed session tracking is already a Recollective feature but we hope to provide additional alerts of risky behaviour (e.g. late night session times, shared IP addresses and the use of VPN software).
If any of these capabilities would be of interest to you, or you have additional ideas to maintain data integrity, please share this feedback with us at https://recollective.com/ideas.

Conclusion
Given the rapidly evolving generative AI industry, I don’t believe researchers should rely on automated detection. Instead, focus on the use of video screening, use variety in your study design and monitor for unnatural responses. Consider asynchronous video capture and live meetings as ways to regularly check in with your participants. And finally, make use of platform features such as pasting blocking and session tracking.
Online qualitative research offers a vast range of possibilities and is even more important now as a source of truth. With proper vigilance in respondent selection and solid research design, platforms like Recollective can deliver those possibilities without compromising on quality. Trusted insights await those willing to learn and adapt to today’s realities.